Lagrangian transforms for signal analysis and machine learning: Mathematical and computational modeling techniques play a crucial role in advancing research in biomedical and clinical sciences. As the problems being tackled become increasingly complex, machine learning methods such as deep learning have emerged as a leading approach for analyzing complex image data. Although the results of deep learning are often impressive on specific datasets, its limitations with respect to accuracy, complexity, the need for large datasets, energy consumption, robustness, and interpretability suggest that further improvements are necessary to mitigate the risks of stagnation and ensure the development of safe and reliable methods for biomedical applications. In this talk I will describe a new, optimal transport-based, image representation framework that enables users to solve regression and machine learning problems more easily, significantly enhancing the impact of deep and other machine learning techniques in a variety of predictive modeling tasks.
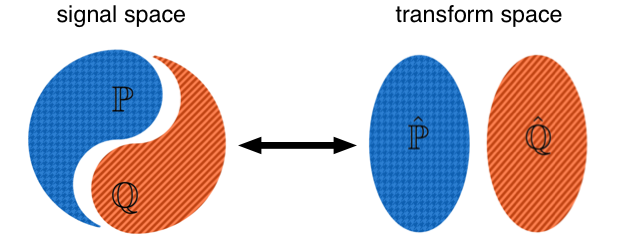
- Project page
- Software: pytranskit
- Invariance encoding in sliced Wasserstein space, 2023 Pattern Rec. Paper
- The signed cumulative distribution transform (SCDT). 2022 AIMS FOD Paper
- End-to-end Signal Classification in SCDT Space, 2022 preprint
- Illumination invariant face recognition using optimal transport, 2022 Preprint
- R-CDT subspace models for image classification, JMIV 21, preprint
- Cumulative Distribution Transform: paper (ACHA 2018)
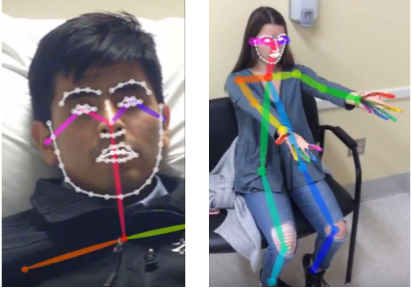
Biometrics and stroke : Stroke is a leading cause of disability worldwide. Early recognition and treatment of stroke decreases the mortality and possibility of severe injury. A significant barrier to accurate diagnosis in neurological disease is that visualized deficits such as eye movement, facial weakness or limb incoordination can be difficult to quantify. Together with collaborators from Neurology at UVa, we are collecting quantitative data from a large patient cohort, as well as controls, and are developing biometric intelligent systems to more objectively quantify signs of stroke and ultimately deliver a low cost, early stroke risk assessment pervasive technology.
- Project page
- Camera-based eye movement assessment. 2023 IEEE JBHI paper
- Quantifying Eye Movements to Augment Stroke Diagnosis With a Non-Calibrated Eye-Tracker. 2022 IEEE TBME paper
- Human vs. Machine Learning Based Detection of Facial Weakness Using Video Analysis. 2022 Frontiers in Neurology paper
- Video-based facial weakness anaysis. 2021 IEEE TBME paper
- Facial weakness analysis and quantification of static images. 2020 IEEE JBHI paper
- Pathological facial weakness detection using computational image analysis, ISBI, 2018. paper
- Robust efficient estimation of heart rate pulse from video. Biomedical Optics Express 2014. paper
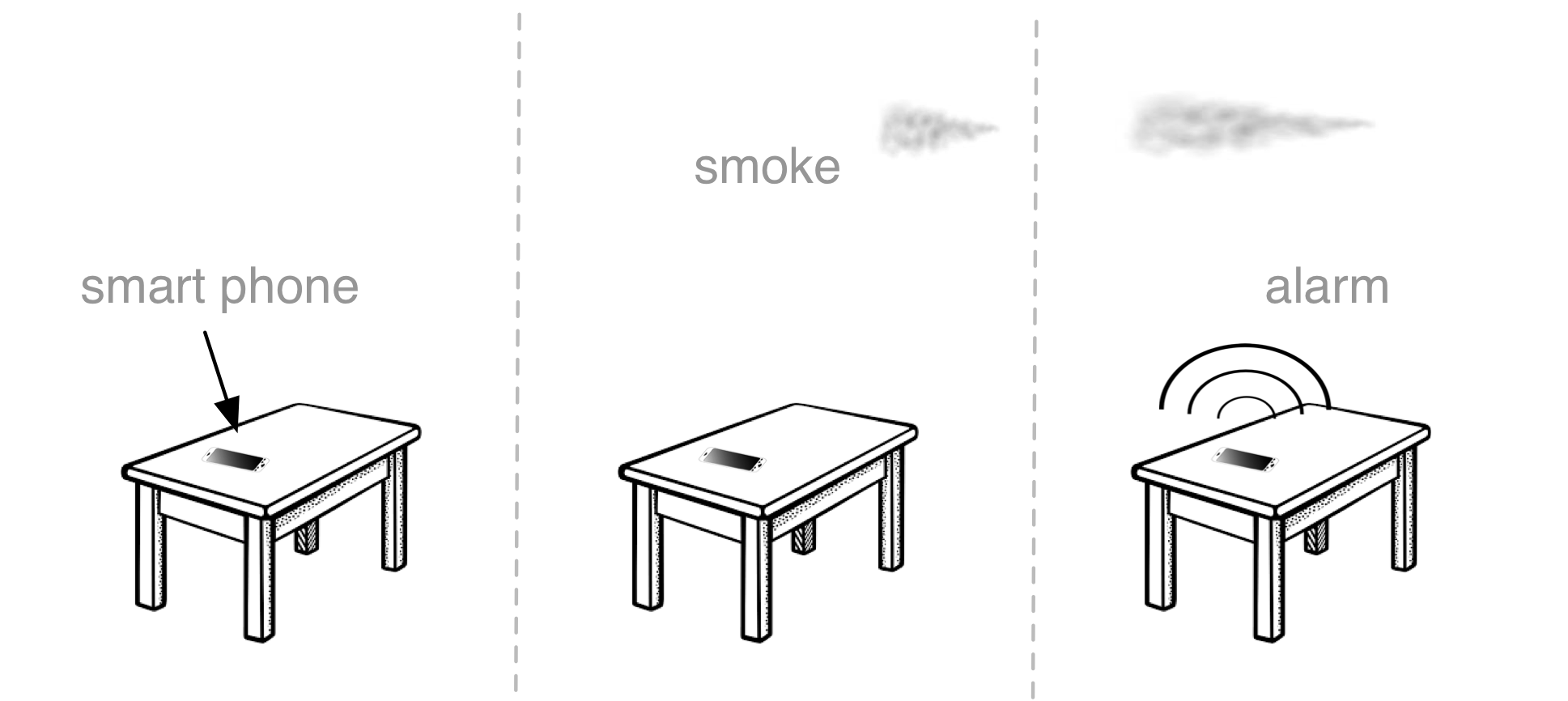
SmokeDetective: Annually in the US, roughly 1 in every 330 households reported a home fire. There is a house fire every 98 seconds and a death once every three hours. Even though over 7 billion in property damage happens every year, 30% of smoke alarms do not work when tested, and smoke alarms are not sounded in 47% of house fires. We are working on algorithms to render any camera enabled smart device (e.g. cell phone, tablet, computer, nanny cam, etc.) into a working smoke detector thus delivering constant, ubiquitous smoke detection and monitoring.
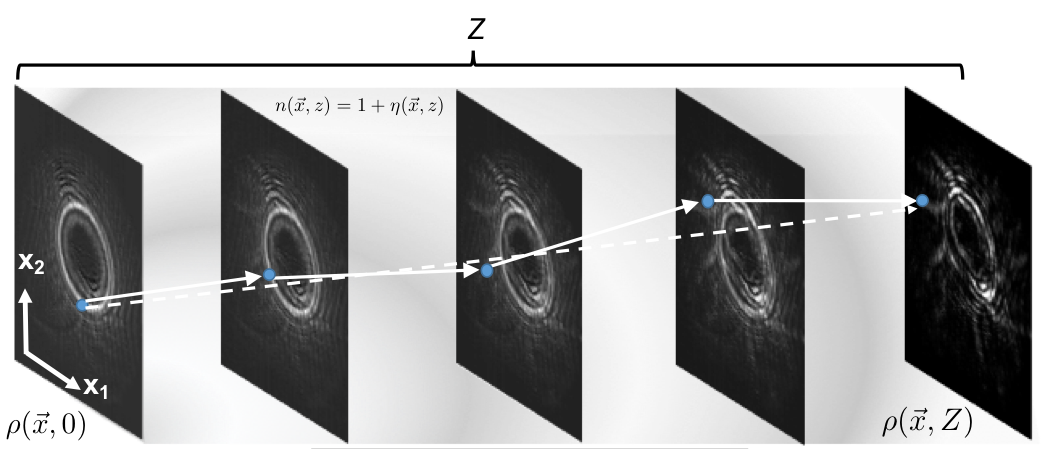
Wave propagation, reduced order modeling, and system identification: In collaboration with colleagues at the Naval Research Laboratory, we're developing new methods for system identification and signal estimation for nonlinear systems. The modeling framework relies on the observation that Lagrangian techniques can efficiently represent wave propagation phenomena, yielding a new framework to solve signal processing (e.g. estimation and detection) and system identification problems. To date we have used the technique to come up with optical image formation models in the presence of turbulence, solve nonlinear signal estimation problems, and estimate PDE/system parameters from signal readouts. a new turbulence image formation model which can be used in practical applications. The modeling approach combines stochastic processes with fluid mechanics approaches and is being applied to inverse problems, communications, and target recognition.
- Transport wave propagation model for for partially coherent polarization-gradient vector beams. JOSA A, 2023. paper
- Transport-based pattern recognition versus deep neural networks in underwater OAM communications. JOSA A, 2021/ paper
- Parametric Signal Estimation Using the Cumulative Distribution Transform. IEEE TSP 2020. paper
- Transport model for beam broadening in turbulence. Modern optics, 2019. paper
- Transport model of atmospheric turbulence, Applied Optics, 2018. paper
- OAM demultipliexing using optimal transport, Optics Express, 2018. paper
Cytometry, High Content Screening, and Digital pathology: Digital microscopy is helping revolutionize cancer research, diagnosis, and treatment. Quantitative image analysis methods can help extract objective quantitative information from large quantities of cells. This information can in turn be used to build predictive models to describe effects of perturbations (e.g. drugs), as well as for aiding diagnosis and prognosis from histology/cytology images. Transport processes underlie the physics of how cells grow, move, and divide. Therefore, we are developing mathematical methods for modeling the information content in microscopy images of cells using these and other techniques with the end goal of providing more accurate, understandable, efficient and robust processing techniques for mining the information content of images of cells.
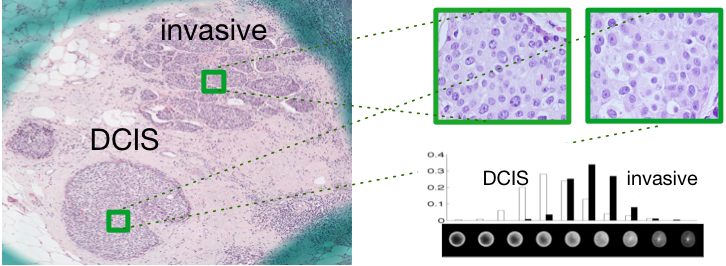
- Transport-based morphometry of nuclear structure in cancer, 2023 preprint
- Effects of COVID‐19 vaccinations on platelets, Cytometry A 2023 paper
- Single-cell profiling reveals high levels of circulating platelet aggregates in patients with COVID-19, Nat. Comm. 2021 paper
- Nuclear chromatin changes in melanoma. paper
- Diagnosing mesothelioma from cytology, Cytometry A, 87(4), 326-333, 2015. pdf
- Nuclear morphology in thyroid follicular lesions. Medical Image Analysis, 2014. link
- Transport-based morphometry of cells, PNAS 2014. pdf , publisher , software
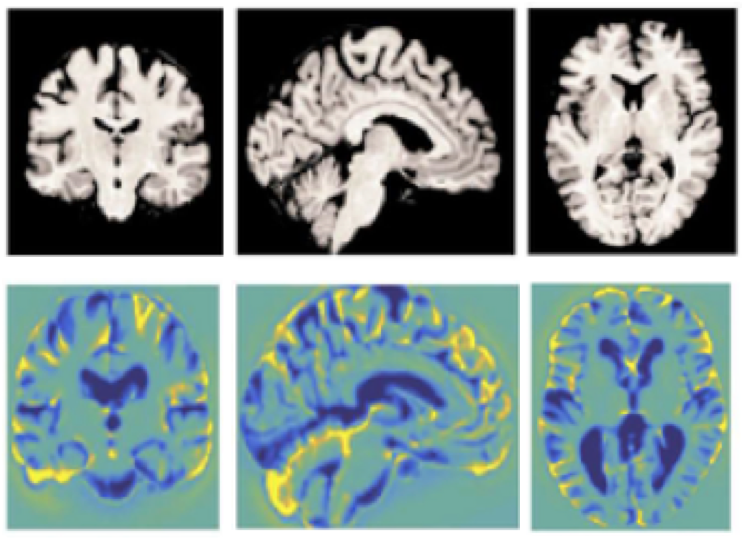
Medical image analysis: Image-based models of structure-function relationships can greatly facilitate our understanding of living systems. Based on a new signal transformation being developed (see here for more information) our group is developing a new approach for quantifying the structure (i.e. shape, texture information) of biological forms seamlessly from image data. The new approach is ideally suited for forms which are associated with transport processes, though it can be used more generally. It has been used to model structure-function relationships and build predictive models of cancer from nuclear structure, knee osteoarthritis from MRI data, reaction time in concussed patients from diffusion MRI data, and others.
- Transport-based morphometry of nuclear structure in cancer, 2023 preprint
- Cardiorespiratory fitness in reducing brain tissue loss caused by ageing, 2021 paper .
- Pre-symptomatic early detection of osteoarthritis from knee MRIs. PNAS, 2020. paper
- Discovery and visualization of structural biomarkers from MRI using transport-based morphometry, Neuroimage 2018. preprint
- Intensity-based nonrigid image registration, IEEE TMI 2003. paper